ICLR 2024 Workshop on
Representational Alignment
(Re-Align)
May 11th, 2024
ICLR 2024 in Vienna, Austria
News
About
The question of What makes a good representation? in machine learning can be addressed in one of several ways: By evaluating downstream behavior, by inspecting internal representations, or by characterizing a system’s inductive biases. Each of these methodologies involves measuring the alignment of an artificial intelligence (AI) system to a ground truth system (usually a human or a population of humans) at some level of analysis (be it behavior, internal representation, or something in between). However, despite this shared goal, the machine learning, neuroscience, and cognitive science communities that study alignment among artificial and biological intelligence systems currently lack a shared framework for conveying insights across methodologies and disciplines.
This workshop aims to bridge this gap by defining, evaluating, and understanding the implications of representational alignment among biological & artificial systems. We invite researchers across the machine learning, neuroscience, and cognitive science communities to contribute to this discussion in the form of invited talks, contributed papers, and structured discussions that address questions such as:
- How can we measure representational alignment among biological and artificial intelligence (AI) systems?
- Can representational alignment tell us if AI systems use the same strategies to solve tasks as humans do?
- What are the consequences (positive, neutral, and negative) of representational alignment?
- How does representational alignment connect to behavioral alignment and value alignment, as understood in AI safety and interpretability & explainability?
- How can we increase (or decrease) representational alignment of an AI system?
- How does the degree of representational alignment between two systems impact their ability to compete, cooperate, and communicate?
In collaboration with other researchers, the organizers have prepared a position paper (📄 paper) that collects prior work and highlights key issues on the topic of representational alignment. A concrete goal of the workshop is to expand this paper with any new insights generated during the workshop.
Invited speakers
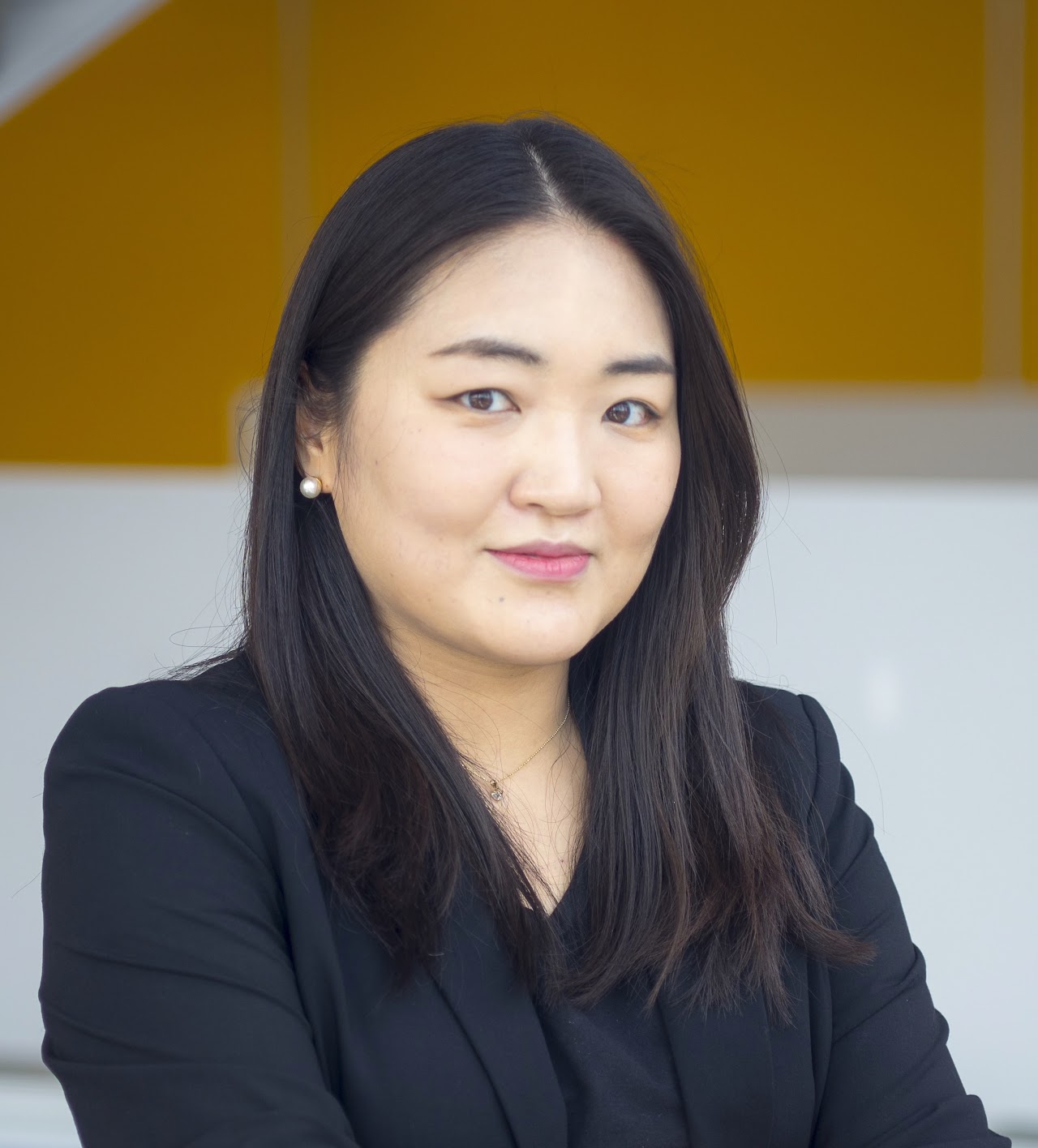
SueYeon Chung
New York University
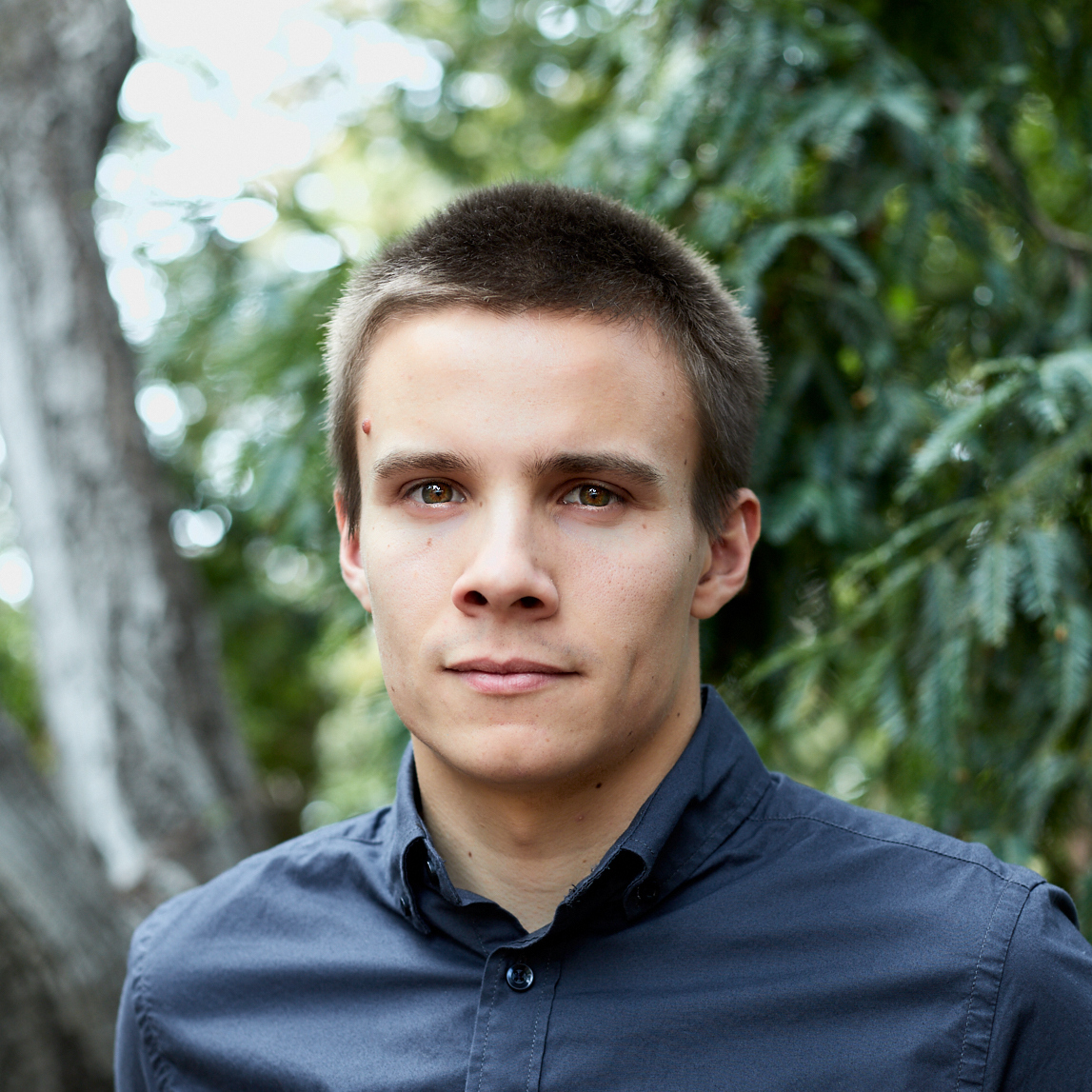
Andrew Lampinen
Google DeepMind
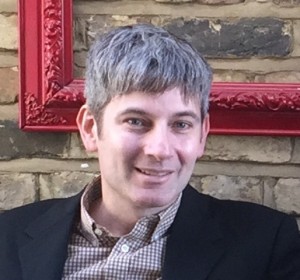
Bradley Love
University College London
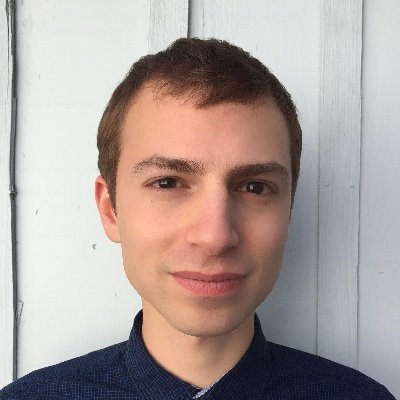
Simon Kornblith
Anthropic
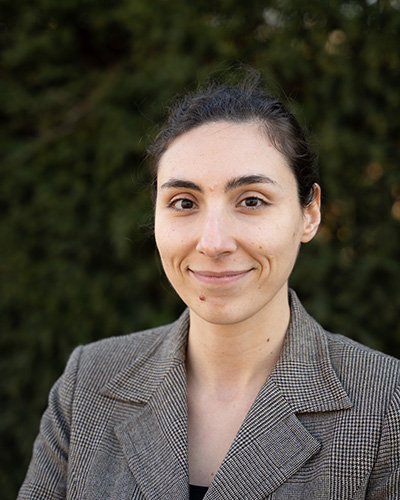
Mariya Toneva
Max Planck Institute for Software Systems
Organizers
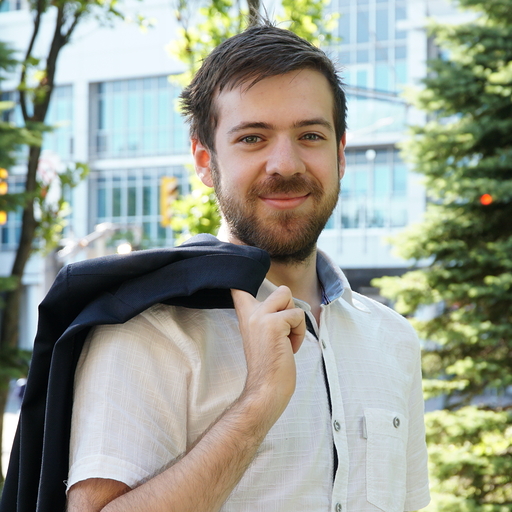
Ilia Sucholutsky
Princeton University
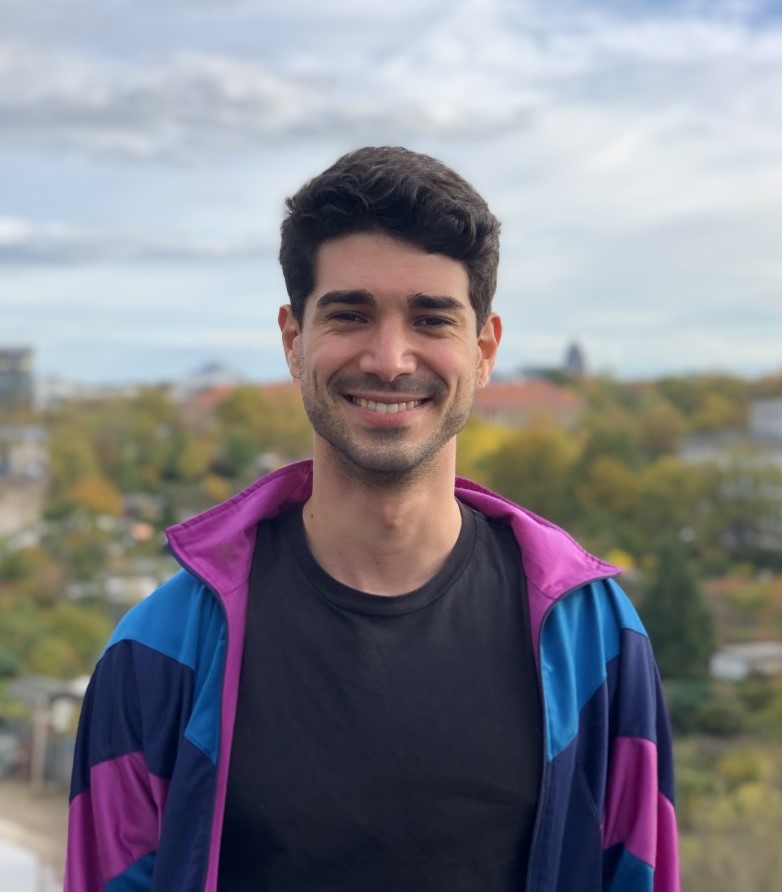
Lukas Muttenthaler
TU Berlin
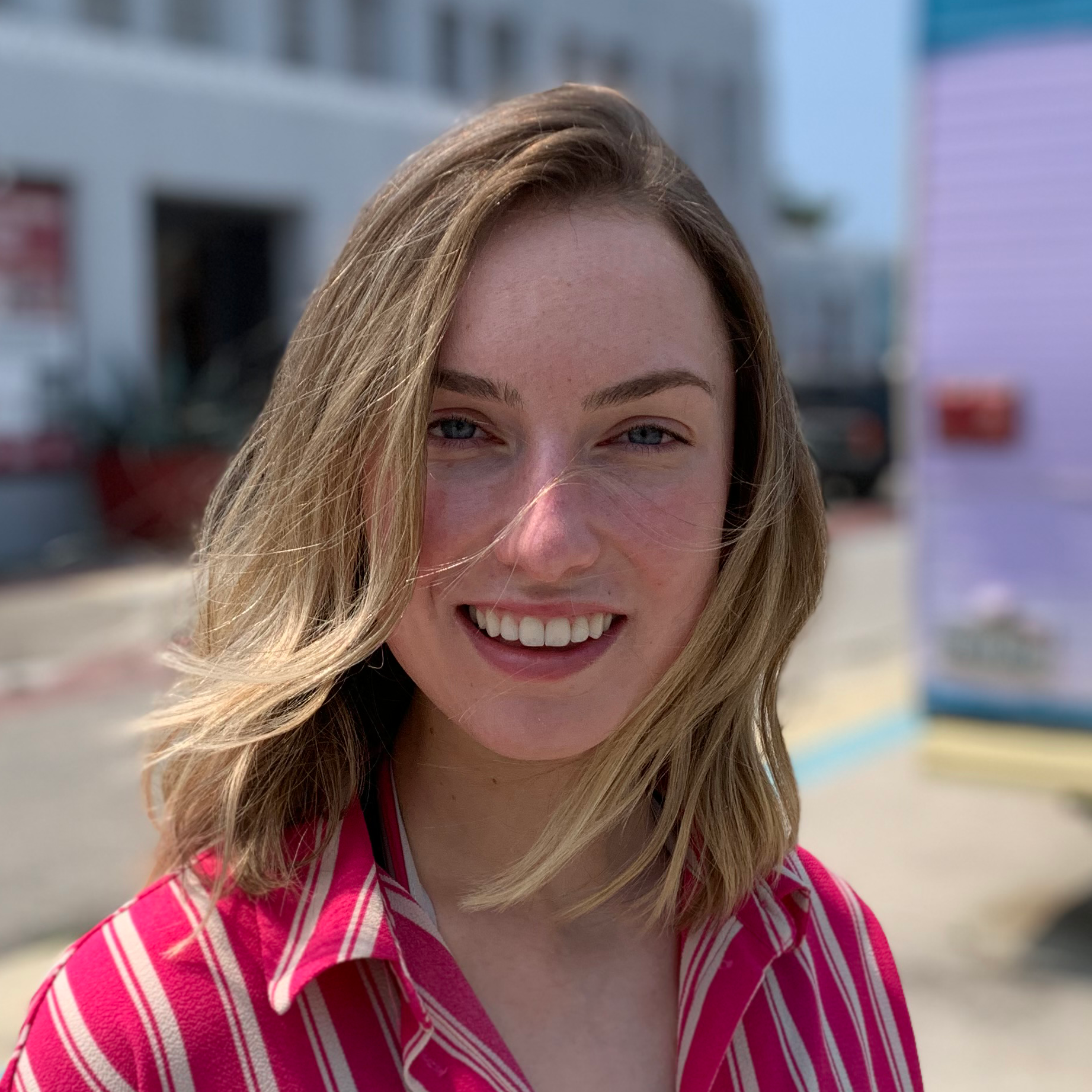
Erin Grant
University College London
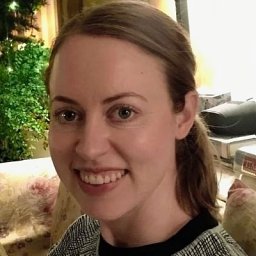
Katherine Hermann
Google DeepMind
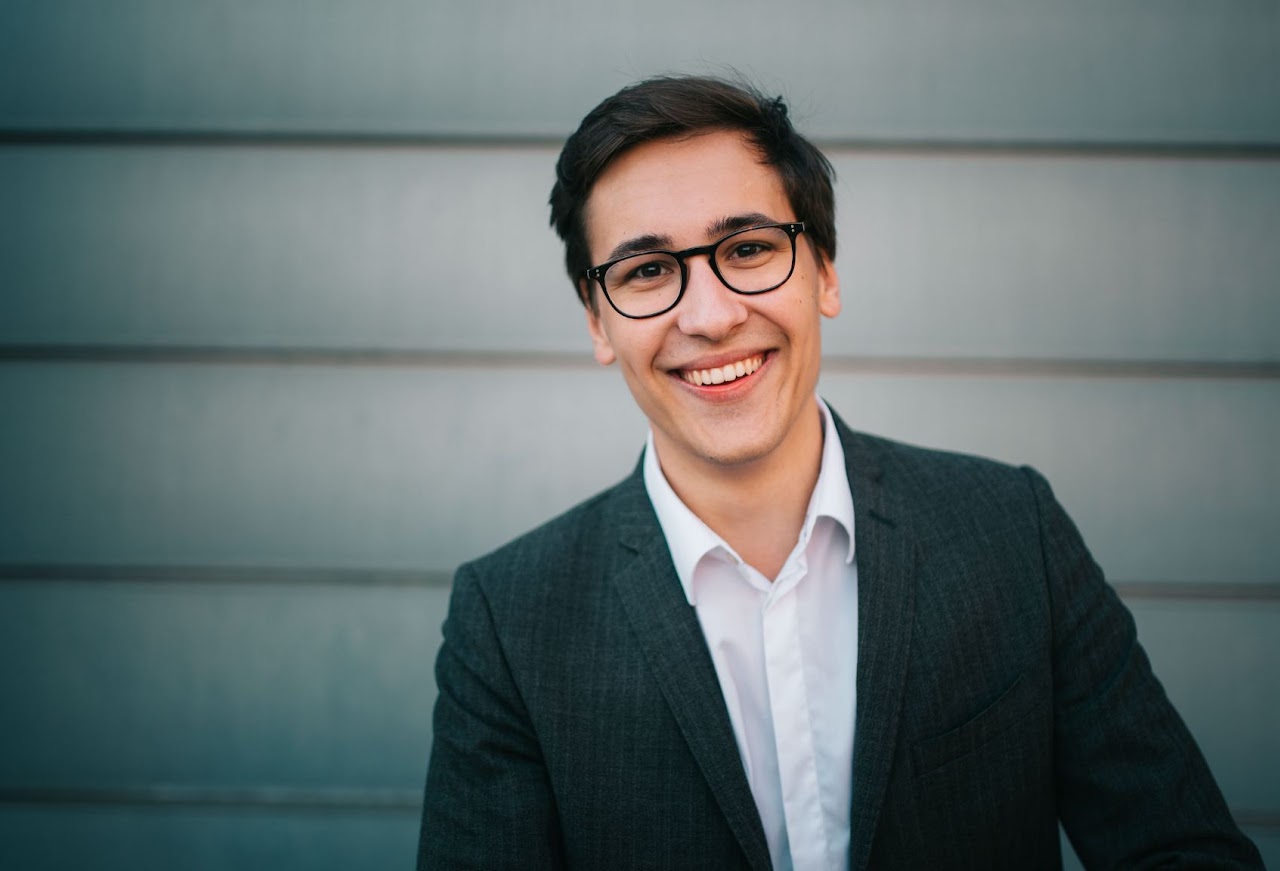
Jascha Achterberg
University of Cambridge
Program committee
We thank the following reviewers for providing thorough and constructive feedback on submissions to the workshop: